Mixtile เป็นบริษัทที่พัฒนาฮาร์ดแวร์โซลูชั่นสำหรับงานหลายๆด้านทั้ง IoT, AI และ Industrial gateway ซึ่งทาง Mixtile ได้ส่งผลิตภัณฑ์ที่ชื่อว่า Mixtile Blade 3 ซึ่งเป็นบอร์ดที่ออกแบบมาน่าสนใจมาก เพราะเป็น RK3588 ที่มี RAM สูงถึง 32 GB และยังมีความน่าสนใจอื่น ๆ อีกที่รอการรีวิว น่าสนใจยังไงมาลองติดตามไปด้วยกัน ตั้งแต่แกะกล่อง ,ทดสอบใช้งาน RKNPU จนถึงลองใช้งาน LLM บน Mixtile Blade 3 นี้กันเลยดีกว่า
แกะกล่อง Mixtile Blade 3
ในกล่องพัสดุที่ทาง Mixtile ส่งมาให้ประกอบด้วยกล่องกระดาษสองกล่อง กล่องแรกคือบอร์ด Mixtile Blade 3 และกล่องที่สองคือ Mixtile Blade 3 Case
เรามาแกะกล่องแรกคือบอร์ด Mixtile Blade 3 กันก่อน เมื่อแกะกล่องก็จะพบกับบอร์ดที่ค่อนข้างมีน้ำหนักเลย พอหยิบมาดูก็พบว่าเพราะมีการใส่ Heat sink ขนาดใหญ่เท่ากับบอร์ดไว้ด้านล่างเพื่อระบายความร้อนจาก RK3588 โดยไม่ต้องใช้พัดลม
เมื่อมาดูอุปกรณ์เชื่อมต่อก็พบว่า มี HDMI 2 ช่องสำหรับเป็น input และ output, Ethernet จำนวน 2 ช่องเช่นเดียวกัน, USB Type-C สองช่อง, GPIO 30 ขา, มีช่องคอนเน็คเตอร์แบบ U.2(SFF-8639) สำหรับต่อกับอุปกรณ์ที่เป็น PCIe/NVMe, คอนเน็คเตอร์ Mini-PCIe, คอนเน็คเเตอร์ MIPI-CSI, ซ็อกเก็ต Micro SD, คอนเน็คเตอร์ต่อพัดลมระบายความร้อน และคอนเน็คเตอร์สำหรับต่อ UART เพื่อ debug
จากนั้นเรามาแกะกล่องของ Mixtile Blade 3 Case กันต่อก็พบว่าเป็นเคสอลูมิเนียมแบบ CNC ที่มาพร้อมกับ adaptor สำหรับต่อเข้ากับคอนเน็คเตอร์ U.2 เป็น M.2 NVMe และปุ่มเปิดปิดรวมถึงไฟ LED บอกสถานะการทำงาน
โดยเราจะมาลองประกอบบอร์ด Mixtile Blade 3 เข้ากับเคส กันต่อไป โดยขั้นแรกที่ต้องทำคือการถอด heat-sink เดิมออกก่อน จากนั้นทำการประกอบ adaptor เข้ากับบอร์ดและใส่ลงในเคส ปิดท้ายด้วยการปิดฝาพร้อมด้วย silicon thermal pad เพื่อฝาปิดนี้จะทำหน้าที่ระบายความร้อนจากบอร์ดมายังเคสทั้งชิ้น
ซึ่งจะพบว่าไม่มี power adaptor มาด้วยการทำงานของบอร์ด Mixtile Blade 3 ต้องการ USB-C ที่สนับสนุนมาตรฐาน PD2.0/PD3.0 จึงจะทำงานได้
คุณสมบัติ
- CPU: เป็น Rockchip RK3588 สถาปัตยกรรม Cortex-A76/A55 แบบ 8 คอร์
- NPU: สูงสุด 6 TOPS
- Memory:
- รองรับ RAM สูงสุดที่ 32 GB แบบ LPDDR4/LPDDR5
- การเก็บข้อมูลแบบ eMMC ความจุสูงสุด 256 GB
- HDMI interface:
- HDMI 2.1 output (8K @ 60 FPS or 4K @ 120 FPS)
- HDMI 2.0 input (4K @ 60 FPS)
- Video encoder: รองรับการเข้ารหัสวิดีโอแบบ H.264/H.265 ความละเอียดสูงสุด 8K ที่ 30 FPS
- Video decoder: รองรับการถอดรหัสวิดีโอแบบH.265/H.264/VP9 vความละเอียดสูงสุด 8K ที่ 60 FPS
- Camera Input: รองรับ MIPI-CSI แบบ 4-lane และ USB webcam
- PCIe expansion: Mini-PCIe socket แบบ PCIe Gen 2.1 และรองรับ USB2.0
- Storage expansion:
- PCIe Gen 3 แบบ 4-lane ผ่านคอนเน็คเตอร์แบบ U.2 port
- SATA 3.0 ผ่าน U.2 port และ Micro-SD 3.0 ซ็อกเก็ต
- Ethernet: gigabit Ethernet ports จำนวน 2 ช่องแบบความเร็ว 2.5 Gbps
- USB:
- USB 3.2 Gen 1 Type-C ports จำนวน 2 ช่อง
- รอบรับ DisplayPort 1.4 A
- GPIOs: 30 พิน
- รอบรับ Digital I/O, I²C, USB 2.0, TTL UART, SPI, I²S
- ซอฟต์แวร์สนับสนุน: ติดตั้ง Ubuntu 22.04 มาในเครื่อง
- สนับสนุน Linux distributions อื่นๆ และ Android 12
- การจ่ายหลังงาน: USB1/PD Type-C Port ที่รองรับ USB PD 2.0 protocol
- (ทางเลือก: ไฟดีซี 12 V ผ่านทาง U.2 port)
- ขนาด: Pico-ITX form factor หรือ 100 x 72 มิลลิเมตร
- การใช้พลังงาน: สูงสุด 10 W
- ช่วงอุณหภูมิในการทำงาน 0 ถึง +80°C
การติดตั้ง OS และทดสอบการใช้งานเบื้องต้น
เนื่องจาก Mixtile Blade 3 มีการติดตั้งระบบปฎิบัติการมาให้เป็น Ubuntu 22.04 อยู่แล้วเราจึงสามารถใช้งานได้ทันทีหลังจากบูตเข้าระบบ แต่หากต้องการจะติดตั้งระบบปฎิบัติการใหม่สามารถทำได้เหมือนการติดตั้งซิงเกิ้ลบอร์ดที่ใช้ SoC ของ Rockchip อื่นๆ คือการติดตั้งผ่าน RKDevTool หรือติดตั้งผ่าน Micro SD
เนื่องจาก Mixtile Blade 3 มี USB พอร์ตให้ใช้งานเพียงสองช่อง ช่องแรกต้องใช้ในการจ่ายไฟให้กับบอร์ด ทำให้เราเหลือ USB C เพียงช่องเดียวจึงจำเป็นต้องมี USB C hub ในการเชื่อมต่อกับคีย์บอร์ดและเม้าส์ด้วย
หลังจากบูตจะเป็นหน้าการตั้งค่าแบบทั่วไปของ Ubuntu หลังจากการตั้งค่าเสร็จสิ้น เราจะสามารถเข้ามาใช้งาน Desktop ของ Ubuntu 22.04 ได้ทันที
โดยเมื่อตรวจสอบขนาดของพื้นที่เก็บข้อมูลก็พบว่าเป็น eMMC ขนาดความจุ 128GB และตรวจพบ SSD ขนาด 256GB ที่ต่อเพิ่มเข้าไปด้วย
1 2 3 4 5 6 7 8 9 10 11 12 13 14 15 16 17 18 19 20 21 22 23 24 25 26 27 28 29 |
arnon@arnon-desktop:~$ sudo fdisk -l Disk /dev/ram0: 4 MiB, 4194304 bytes, 8192 sectors Units: sectors of 1 * 512 = 512 bytes Sector size (logical/physical): 512 bytes / 4096 bytes I/O size (minimum/optimal): 4096 bytes / 4096 bytes Disk /dev/mmcblk0: 116.48 GiB, 125069950976 bytes, 244277248 sectors Units: sectors of 1 * 512 = 512 bytes Sector size (logical/physical): 512 bytes / 512 bytes I/O size (minimum/optimal): 512 bytes / 512 bytes Disklabel type: gpt Disk identifier: AE8C1F40-2C40-4F65-AD1F-F7DD7D785383 Device Start End Sectors Size Type /dev/mmcblk0p1 32768 1081343 1048576 512M Linux extended boot /dev/mmcblk0p2 1081344 244277214 243195871 116G Linux filesystem Disk /dev/nvme0n1: 232.89 GiB, 250059350016 bytes, 488397168 sectors Disk model: WD_BLACK SN770 250GB Units: sectors of 1 * 512 = 512 bytes Sector size (logical/physical): 512 bytes / 512 bytes I/O size (minimum/optimal): 512 bytes / 512 bytes Disklabel type: dos Disk identifier: 0x71d948da Device Boot Start End Sectors Size Id Type /dev/nvme0n1p1 2048 488397167 488395120 232.9G 83 Linux |
ในส่วนของหน่วยความจำจะพบว่ามีขนาดความจำอยู่ที่ 32GB ซึ่งถือว่าสูงมากสำหรับ SBC
1 2 3 4 |
arnon@arnon-desktop:~$ free -m total used free shared buff/cache available Mem: 31787 604 30595 5 587 30891 Swap: 2047 0 2047 |
การทดสอบประสิทธิภาพ AI ผ่าน NPU ด้วย RKNPU2
ในการทดสอบประสิทธิภาพ AI ของ Mixtile Blade 3 เราจะทำการทดสอบเทียบกับผลจาก RockChip RK3568 ทีมี NPU ขนาด 0.8 TOPS โดยการทดสอบบนตัวอย่างของ RKNPU2 ในส่วนของ yolov5_demo และ RKNN Benchmark ซึ่งเราเคยทดสอบไว้แล้วกับ youyeeyoo YY3568
โดยหลังจากติดตั้ง RKNN toolkit2 จาก Github เรียบร้อยแล้ว เราจะทำการ compile ตัวอย่างของ YOLO5
1 2 3 4 5 6 7 8 9 10 11 12 13 14 15 16 17 18 19 20 21 22 23 24 25 26 27 28 29 30 31 32 33 34 35 36 37 38 39 40 41 42 43 44 45 46 47 |
arnon@arnon-desktop:~$ cd rknn-toolkit2/rknpu2/examples/rknn_yolov5_demo/ arnon@arnon-desktop:~/rknn-toolkit2/rknpu2/examples/rknn_yolov5_demo$ ./build-linux_RK3588.sh clean -- The C compiler identification is GNU 11.4.0 -- The CXX compiler identification is GNU 11.4.0 -- Detecting C compiler ABI info -- Detecting C compiler ABI info - done -- Check for working C compiler: /usr/bin/aarch64-linux-gnu-gcc - skipped -- Detecting C compile features -- Detecting C compile features - done -- Detecting CXX compiler ABI info -- Detecting CXX compiler ABI info - done -- Check for working CXX compiler: /usr/bin/aarch64-linux-gnu-g++ - skipped -- Detecting CXX compile features -- Detecting CXX compile features - done -- Found OpenCV: /home/arnon/rknn-toolkit2/rknpu2/examples/3rdparty/opencv/opencv-linux-aarch64 (found version "3.4.5") -- Configuring done -- Generating done -- Build files have been written to: /home/arnon/rknn-toolkit2/rknpu2/examples/rknn_yolov5_demo/build/build_linux_aarch64 [ 20%] Building CXX object CMakeFiles/rknn_yolov5_demo.dir/src/preprocess.cc.o [ 20%] Building CXX object CMakeFiles/rknn_yolov5_video_demo.dir/src/main_video.cc.o [ 40%] Building CXX object CMakeFiles/rknn_yolov5_demo.dir/src/main.cc.o [ 40%] Building CXX object CMakeFiles/rknn_yolov5_demo.dir/src/postprocess.cc.o [ 50%] Building CXX object CMakeFiles/rknn_yolov5_video_demo.dir/src/postprocess.cc.o [ 60%] Building CXX object CMakeFiles/rknn_yolov5_video_demo.dir/utils/mpp_decoder.cpp.o [ 70%] Building CXX object CMakeFiles/rknn_yolov5_video_demo.dir/utils/mpp_encoder.cpp.o [ 80%] Building CXX object CMakeFiles/rknn_yolov5_video_demo.dir/utils/drawing.cpp.o [ 90%] Linking CXX executable rknn_yolov5_video_demo [ 90%] Built target rknn_yolov5_video_demo [100%] Linking CXX executable rknn_yolov5_demo [100%] Built target rknn_yolov5_demo Consolidate compiler generated dependencies of target rknn_yolov5_demo [ 40%] Built target rknn_yolov5_demo Consolidate compiler generated dependencies of target rknn_yolov5_video_demo [100%] Built target rknn_yolov5_video_demo Install the project... -- Install configuration: "" -- Installing: /home/arnon/rknn-toolkit2/rknpu2/examples/rknn_yolov5_demo/install/rknn_yolov5_demo_Linux/./rknn_yolov5_demo -- Up-to-date: /home/arnon/rknn-toolkit2/rknpu2/examples/rknn_yolov5_demo/install/rknn_yolov5_demo_Linux/lib/librknnrt.so -- Up-to-date: /home/arnon/rknn-toolkit2/rknpu2/examples/rknn_yolov5_demo/install/rknn_yolov5_demo_Linux/lib/librga.so -- Up-to-date: /home/arnon/rknn-toolkit2/rknpu2/examples/rknn_yolov5_demo/install/rknn_yolov5_demo_Linux/./model/RK3588 -- Up-to-date: /home/arnon/rknn-toolkit2/rknpu2/examples/rknn_yolov5_demo/install/rknn_yolov5_demo_Linux/./model/RK3588/yolov5s-640-640.rknn -- Up-to-date: /home/arnon/rknn-toolkit2/rknpu2/examples/rknn_yolov5_demo/install/rknn_yolov5_demo_Linux/./model/bus.jpg-- Up-to-date: /home/arnon/rknn-toolkit2/rknpu2/examples/rknn_yolov5_demo/install/rknn_yolov5_demo_Linux/./model/coco_80_labels_list.txt -- Installing: /home/arnon/rknn-toolkit2/rknpu2/examples/rknn_yolov5_demo/install/rknn_yolov5_demo_Linux/./rknn_yolov5_video_demo -- Up-to-date: /home/arnon/rknn-toolkit2/rknpu2/examples/rknn_yolov5_demo/install/rknn_yolov5_demo_Linux/lib/librockchip_mpp.so -- Up-to-date: /home/arnon/rknn-toolkit2/rknpu2/examples/rknn_yolov5_demo/install/rknn_yolov5_demo_Linux/lib/libmk_api.so /home/arnon/rknn-toolkit2/rknpu2/examples/rknn_yolov5_demo arnon@arnon-desktop:~/rknn-toolkit2/rknpu2/examples/rknn_yolov5_demo$ |
จากนั้นทำการทดสอบผลการตรวบสอบวัตถุด้วย YOLO5
1 2 3 4 5 6 7 8 9 10 11 12 13 14 15 16 17 18 19 20 21 22 23 24 25 26 27 28 29 30 31 32 33 34 35 36 37 38 39 40 41 42 43 |
arnon@arnon-desktop:~/rknn-toolkit2/rknpu2/examples/rknn_yolov5_demo/install/rknn_yolov5_demo_Linux$ ./rknn_yolov5_demo model/RK3588/yolov5s-640-640.rknn model/man.jpg post process config: box_conf_threshold = 0.25, nms_threshold = 0.45 Loading mode... sdk version: 1.6.0 (9a7b5d24c@2023-12-13T17:31:11) driver version: 0.9.2 model input num: 1, output num: 3 index=0, name=images, n_dims=4, dims=[1, 640, 640, 3], n_elems=1228800, size=1228800, w_stride = 640, size_with_stride=1228800, fmt=NHWC, type=INT8, qnt_type=AFFINE, zp=-128, scale=0.003922 index=0, name=output, n_dims=4, dims=[1, 255, 80, 80], n_elems=1632000, size=1632000, w_stride = 0, size_with_stride=1638400, fmt=NCHW, type=INT8, qnt_type=AFFINE, zp=-128, scale=0.003860 index=1, name=283, n_dims=4, dims=[1, 255, 40, 40], n_elems=408000, size=408000, w_stride = 0, size_with_stride=491520, fmt=NCHW, type=INT8, qnt_type=AFFINE, zp=-128, scale=0.003922 index=2, name=285, n_dims=4, dims=[1, 255, 20, 20], n_elems=102000, size=102000, w_stride = 0, size_with_stride=163840, fmt=NCHW, type=INT8, qnt_type=AFFINE, zp=-128, scale=0.003915 model is NHWC input fmt model input height=640, width=640, channel=3 Read model/man.jpg ... img width = 640, img height = 640 once run use 25.523000 ms loadLabelName ./model/coco_80_labels_list.txt person @ (89 157 258 631) 0.895037 bowl @ (483 221 506 240) 0.679969 bowl @ (395 322 444 343) 0.659576 wine glass @ (570 200 588 241) 0.544585 bowl @ (505 221 527 239) 0.477606 bowl @ (482 322 532 338) 0.458121 wine glass @ (543 199 564 239) 0.452579 cup @ (418 215 437 238) 0.410092 cup @ (385 204 402 240) 0.374592 cup @ (435 212 451 238) 0.371657 bowl @ (613 215 639 239) 0.359605 wine glass @ (557 200 575 240) 0.359143 cup @ (446 211 461 238) 0.358369 spoon @ (255 257 271 313) 0.340807 bottle @ (412 84 432 119) 0.338540 spoon @ (307 267 322 326) 0.318563 spoon @ (324 265 340 332) 0.315867 bottle @ (453 305 466 340) 0.308927 cup @ (526 210 544 239) 0.290318 bottle @ (389 83 411 119) 0.277804 wine glass @ (583 198 602 239) 0.277093 bowl @ (24 359 101 383) 0.275663 oven @ (4 370 168 632) 0.256395 spoon @ (268 262 282 322) 0.252866 bottle @ (434 85 454 118) 0.250721 save detect result to ./out.jpg loop count = 10 , average run 18.620700 ms arnon@arnon-desktop:~/rknn-toolkit2/rknpu2/examples/rknn_yolov5_demo/install/rknn_yolov5_demo_Linux$ |
ซึ่งเมื่อนำค่าที่ได้ไปเปรียบเทียบกับผลลัพธ์จาก RK3568 จะพบความแตกต่างดังนี้
บอร์ด | ทำงานหนึ่งครั้ง | ค่าเฉลี่ย 10 ครั้ง |
---|---|---|
Mixtile Blade 3 | 25.523000 ms | 18.620700 ms |
RK3568 อื่น | 78.917000 ms | 69.709700 ms |
ซึ่งประสิทธิภาพที่วัดได้บอร์ด Mixtile Blade 3 มากกว่า RK3568 มาก โดยใช้เวลาน้อยกว่า RK3568 ที่เคยทดสอบ 3 เท่า คิดออกมาเป็นหน่วย FPS จะอยู่ที่ 54 FPS ซึ่งถือว่าประมวลผลได้เร็วมาก
จากนั้นเราจะมาลองทดสอบประสิทธิภาพของ NPU บน Mixtile Blade 3 ด้วยตัวอย่าง RKNN Benchmark ที่อยู่ใน examples โดยระบุให้ทำการวนทดสอบ 10 ครั้งแล้วหาค่าเฉลี่ย
1 2 3 4 5 6 7 8 9 10 11 12 13 14 15 16 17 18 19 20 21 22 23 24 25 26 27 28 29 30 31 32 33 34 35 36 37 38 39 40 41 42 43 44 45 46 47 48 49 50 51 52 53 |
arnon@arnon-desktop:~/rknn-toolkit2/rknpu2/examples/rknn_benchmark/install/rknn_benchmark_Linux$ ./rknn_benchmark /home/arnon/rknn-toolkit2/rknpu2/examples/rknn_yolov5_demo/install/rknn_yolov5_demo_Linux/model/RK3588/yolov5s-640-640.rknn /home/arnon/rknn-toolkit2/rknpu2/examples/rknn_yolov5_demo/install/rknn_yolov5_demo_Linux/model/man.jpg rknn_api/rknnrt version: 1.6.0 (9a7b5d24c@2023-12-13T17:31:11), driver version: 0.9.2 total weight size: 7312128, total internal size: 7782400 total dma used size: 24784896 model input num: 1, output num: 3 input tensors: index=0, name=images, n_dims=4, dims=[1, 640, 640, 3], n_elems=1228800, size=1228800, w_stride = 640, size_with_stride=1228800, fmt=NHWC, type=INT8, qnt_type=AFFINE, zp=-128, scale=0.003922 output tensors: index=0, name=output, n_dims=4, dims=[1, 255, 80, 80], n_elems=1632000, size=1632000, w_stride = 0, size_with_stride=1638400, fmt=NCHW, type=INT8, qnt_type=AFFINE, zp=-128, scale=0.003860 index=1, name=283, n_dims=4, dims=[1, 255, 40, 40], n_elems=408000, size=408000, w_stride = 0, size_with_stride=491520, fmt=NCHW, type=INT8, qnt_type=AFFINE, zp=-128, scale=0.003922 index=2, name=285, n_dims=4, dims=[1, 255, 20, 20], n_elems=102000, size=102000, w_stride = 0, size_with_stride=163840, fmt=NCHW, type=INT8, qnt_type=AFFINE, zp=-128, scale=0.003915 custom string: Warmup ... 0: Elapse Time = 21.00ms, FPS = 47.63 1: Elapse Time = 20.88ms, FPS = 47.89 2: Elapse Time = 20.89ms, FPS = 47.87 3: Elapse Time = 20.11ms, FPS = 49.71 4: Elapse Time = 15.84ms, FPS = 63.14 Begin perf ... 0: Elapse Time = 15.82ms, FPS = 63.23 1: Elapse Time = 15.87ms, FPS = 63.01 2: Elapse Time = 15.87ms, FPS = 63.00 3: Elapse Time = 15.69ms, FPS = 63.72 4: Elapse Time = 15.89ms, FPS = 62.95 5: Elapse Time = 15.78ms, FPS = 63.36 6: Elapse Time = 15.92ms, FPS = 62.83 7: Elapse Time = 15.83ms, FPS = 63.17 8: Elapse Time = 15.88ms, FPS = 62.98 9: Elapse Time = 15.88ms, FPS = 62.98 Avg Time 15.84ms, Avg FPS = 63.123 Save output to rt_output0.npy Save output to rt_output1.npy Save output to rt_output2.npy ---- Top5 ---- 0.984223 - 16052 0.984223 - 16132 0.984223 - 16212 0.984223 - 560644 0.984223 - 560724 ---- Top5 ---- 0.996078 - 280970 0.996078 - 281010 0.996078 - 281011 0.992157 - 280769 0.992157 - 280770 ---- Top5 ---- 0.998327 - 70225 0.998327 - 70244 0.998327 - 70245 0.994412 - 36225 0.994412 - 36244 |
ข้อมูลที่ได้จากการทดสอบ benchmark จะพบว่าค่า FPS เฉลี่ยจะอยู่ที่ 63.123 เฟรมต่อวินาที แสดงให้เห็นถึงประสิทธิภาพของบอร์ด Mixtile Blade 3 ว่าเหมาะสมกับการนำมาใช้งานเป็น Edge คอมพิวเตอร์ในงาน AI ด้าน image processing หรือ machine learning
และเพื่อให้เห็นถึงประสิทธิภาพของ Mixtile Blade 3 มากขึ้น เราจะทดสอบ Yolo5 จากกล้องกันบ้าง โดยจะทดสอบประมวลผลวิดีโอที่สตรีมาแบบ RTSP เหมือนกันงานประมวลผล Edge AI ที่ใช้กันทั่วไป โดยเริ่มจากสร้าง RTSP server บนตัวของ Mixtile Blade 3 เองเลยโดยการติดตั้งบน Github
และทำการตั้งค่าในไฟล์ mediamtx.yml ให้สตรีมภาพจากกล้องเว็บแคมที่ความละเอียด 640×640 การเข้ารหัสเป็น h.264
1 2 3 4 |
paths: cam: runOnInit: ffmpeg -f v4l2 -framerate 24 -video_size 640x640 -i /dev/video1 -vcodec h264 -f rtsp rtsp://localhost:$RTSP_PORT/$MTX_PATH runOnInitRestart: yes |
จากนั้นทดสอบการทำงานด้วยคำสั่ง
1 |
./rknn_yolov5_video_demo model/RK3588/yolov5s-640-640.rknn rtsp://127.0.0.1:8554/cam 264 |
ข้อมูลการ detect จะแสดงใน log file เนื่องจากไม่ได้ใช้ open CV ในการทดสอบ จึงทำเป็นแค่การวาดกรอบรอบวัตถุที่ตรวจพบเท่านั้น
1 2 3 4 5 6 7 8 9 10 11 12 13 14 15 16 17 18 19 20 21 22 23 24 25 26 27 28 29 30 31 32 |
resize with RGA! once run use 18.728000 ms post process config: box_conf_threshold = 0.25, nms_threshold = 0.45 cat @ (515 420 640 479) 0.692027 person @ (26 5 520 469) 0.661832 cup @ (375 199 456 272) 0.629220 laptop @ (277 333 424 405) 0.405269 knife @ (438 159 510 267) 0.156645 chn 0 size 5389 qp 27 time_gap=9on_track_frame_out ctx=0xffffc6769500 decoder=0xaaaade2ab060 receive packet size=27460 on_track_frame_out ctx=0xffffc6769500 decoder=0xaaaade2ab060 receive packet size=36555 decoder require buffer w:h [640:480] stride [640:480] buf_size 614400 pts=0 dts=0 get one frame 1708769692278 data_vir=0xffff88022000 fd=66 input image 640x480 stride 640x480 format=2560 resize with RGA! once run use 23.140000 ms post process config: box_conf_threshold = 0.25, nms_threshold = 0.45 person @ (18 9 519 468) 0.647593 cat @ (518 417 640 479) 0.612846 cup @ (371 201 455 271) 0.451749 spoon @ (445 162 509 246) 0.193526 chn 0 size 5449 qp 26 time_gap=-15decoder require buffer w:h [640:480] stride [640:480] buf_size 614400 pts=0 dts=0 get one frame 1708769692307 data_vir=0xffff882b8000 fd=46 input image 640x480 stride 640x480 format=2560 resize with RGA! once run use 18.781000 ms post process config: box_conf_threshold = 0.25, nms_threshold = 0.45 cat @ (518 415 640 479) 0.775714 person @ (24 3 512 473) 0.634580 cup @ (370 201 453 272) 0.592880 knife @ (439 159 507 267) 0.154147 chn 0 size 5585 qp 26 time_gap=9on_track_frame_out ctx=0xffffc6769500 |
จากคลิปวิดีโอแสดงให้เห็นถึงประสิทธิภาพการประมวลที่ดี ได้อัตราเฟรมเรตที่สูง ภาพลื่นไหลไม่มีการกระตุกของภาพให้เห็นแต่อย่างใด
ทดสอบประสิทธิภาพการใช้งาน LLM บน Mixtile Blade 3
จากนั้น เราจะมาทดสอบการใช้งาน LLM บน Mixtile Blade 3 กันต่อ โดยต้องเล่าก่อนว่าบนบอร์ด Mixtile Blade 3 ใช้ SoC RK3588 จึงมี GPU เป็น Mali G610 ซึ่งมีหลายคนได้ทดสอบใช้งาน LLM บน Mali G610 กันแล้ว
โดยในตอนแรกเราได้พยายามทดสอบตามคำแนะนำบน Github แต่ไม่ประสบผลสำเร็จ แต่สุดท้ายก็พบกับบทความแนะนำการทดลองใช้งาน LLM บน RK3588 เลยสามารถทดสอบได้โดยตัว docker มีการสร้างมาโดยใช้โมเดล RedPajama-INCITE-Chat-3B-v1-q4f16_1 และ Llama-2-7b-chat-hf-q4f16_1 แต่เราวางแผนที่จะทำการทดสอบด้วยโมเดล Llama-2-13b-chat-hf-q4f16_1 เพิ่มเติมด้วย เพื่อดูประสิทธิภาพว่าที่ 13 พันล้าน parameter จะใช้ RAM ในการทำงานเยอะแค่ไหน และการใช้งานจริงสามารถทำได้หรือไม่
ทดสอบกับโมเดล RedPajama-INCITE-Chat-3B-v1-q4f16_1
เริ่มต้นด้วยการสั่งเริ่มการทำงานของ docker
1 |
docker run --rm -it --privileged docker.io/milas/mlc-llm:redpajama-3b |
และทำการถามด้วย prompt “Explain why free electrons in an insulator cannot jump over the energy gap to the conduction band”
1 2 3 4 5 6 7 8 9 10 11 12 13 14 15 16 17 18 19 20 21 22 23 24 25 26 27 |
arnon@arnon-desktop:~$ docker run --rm -it --privileged docker.io/milas/mlc-llm:redpajama-3b Use MLC config: "/mlc-llm/dist/prebuilt/RedPajama-INCITE-Chat-3B-v1-q4f16_1/mlc-chat-config.json" Use model weights: "/mlc-llm/dist/prebuilt/RedPajama-INCITE-Chat-3B-v1-q4f16_1/ndarray-cache.json" Use model library: "/mlc-llm/dist/prebuilt/lib/RedPajama-INCITE-Chat-3B-v1-q4f16_1-mali.so" You can use the following special commands: /help print the special commands /exit quit the cli /stats print out the latest stats (token/sec) /reset restart a fresh chat /reload [local_id] reload model `local_id` from disk, or reload the current model if `local_id` is not specified Loading model... arm_release_ver of this libmali is 'g6p0-01eac0', rk_so_ver is '7'. Loading finished Running system prompts... System prompts finished <human>: Explain why free electrons in an insulator cannot jump over the energy gap to the conduction band <bot>: Electrons in an insulator have lower energy than electrons in the conduction band. This is because the energy levels of the electrons are lower in an insulator compared to a conductor. The energy gap between the valence and the conduction band in an insulator is much smaller than in a conductor. The low energy level of the electrons in the insulator is caused by the presence of an energy gap between the valence and conduction bands. Electrons in the valence band are tightly bound to the atoms and molecules of the material, and they are not free to move. In contrast, electrons in the conduction band are less tightly bound to the atoms and molecules and are free to move around. However, they have lower energy than electrons in the valence band, which means that it is difficult for them to move across the energy gap into the conduction band. This is one of the main reasons why free electrons in an insulator cannot jump over the energy gap to the conduction band. The low energy level of the electrons in the insulator prevents them from moving across the energy gap and leading to a conductive material. <human>: /stats prefill: 4.6 tok/s, decode: 5.1 tok/s |
ซึ่งในขณะที่ระบบทำการประมวลผลโมเดล RedPajama-INCITE-Chat-3B-v1-q4f16_1 จะใช้ RAM อยู่ที่ 3.9G
ทดสอบกับโมเดล Llama-2-7b-chat-hf-q4f16_1
เริ่มต้นด้วยการสั่งเริ่มการทำงานของ docker
1 |
docker run --rm -it --privileged docker.io/milas/mlc-llm:llama2-7b |
และทำการถามด้วย prompt “Explain why free electrons in an insulator cannot jump over the energy gap to the conduction band”
1 2 3 4 5 6 7 8 9 10 11 12 13 14 15 16 17 18 19 20 21 22 23 |
arnon@arnon-desktop:~$ docker run --rm -it --privileged docker.io/milas/mlc-llm:llama2-7b Use MLC config: "/mlc-llm/dist/prebuilt/Llama-2-7b-chat-hf-q4f16_1/mlc-chat-config.json" Use model weights: "/mlc-llm/dist/prebuilt/Llama-2-7b-chat-hf-q4f16_1/ndarray-cache.json" Use model library: "/mlc-llm/dist/prebuilt/lib/Llama-2-7b-chat-hf-q4f16_1-mali.so" You can use the following special commands: /help print the special commands /exit quit the cli /stats print out the latest stats (token/sec) /reset restart a fresh chat /reload [local_id] reload model `local_id` from disk, or reload the current model if `local_id` is not specified Loading model... arm_release_ver of this libmali is 'g6p0-01eac0', rk_so_ver is '7'. Loading finished Running system prompts... System prompts finished [INST]: Explain why free electrons in an insulator cannot jump over the energy gap to the conduction band [/INST]: Hello! I'm here to help you with your question. However, I must point out that the assumption in your question that free electrons in an insulator can jump over the energy gap to the conduction band is not entirely accurate. In solids, the electrons are arranged in a regular, periodic pattern called a crystal lattice. The energy gap, also known as the bandgap, is the energy difference between the valence band and the conduction band. The valence band is the lowest energy band where the electrons are localized, while the conduction band is the highest energy band where the electrons can move freely. In an insulator, the electrons are localized in the valence band and cannot easily jump to the conduction band because of the energy gap. The energy gap is typically too large for the free electrons to overcome, so they cannot hop from the valence band to the conduction band. I hope this clears up any confusion! If you have any further questions or need more clarification, please feel free to ask. [INST]: /stats prefill: 4.8 tok/s, decode: 2.8 tok/s |
ซึ่งในขณะที่ระบบทำการประมวลผลโมเดล Llama-2-7b-chat-hf-q4f16_1 จะใช้ RAM อยู่ที่ 6.7G
ทดสอบกับโมเดล Llama-2-13b-chat-hf-q4f16_1
ซึ่งในการทดสอบโมเดลนี้เราจะใช้ docker.io/milas/mlc-llm:redpajama-3b แล้วทำการนำไฟล์ที่เกี่ยวข้องกับโมดเดล Llama-2-13b-chat-hf-q4f16_1 ไปวางไว้บน docker ก่อนที่จะทำการ reload โมเดล ใหม่ และทำการถามด้วย prompt “Explain why free electrons in an insulator cannot jump over the energy gap to the conduction band”
1 2 3 4 5 6 7 8 9 10 11 12 13 14 15 16 17 18 19 20 21 22 23 24 25 26 27 28 29 30 |
arnon@arnon-desktop:~/mlc-llm$ docker attach 4695123cf100 hello <bot>: Hello! How may I help you? <human>: /help You can use the following special commands: /help print the special commands /exit quit the cli /stats print out the latest stats (token/sec) /reset restart a fresh chat /reload [local_id] reload model `local_id` from disk, or reload the current model if `local_id` is not specified <human>: /reload Llama-2-13b-chat-hf-q4f16_1 Use MLC config: "/mlc-llm/dist/prebuilt/Llama-2-13b-chat-hf-q4f16_1/mlc-chat-config.json" Use model weights: "/mlc-llm/dist/prebuilt/Llama-2-13b-chat-hf-q4f16_1/ndarray-cache.json" Use model library: "/mlc-llm/dist/prebuilt/lib/Llama-2-13b-chat-hf-q4f16_1-mali.so" Loading model... Loading finished [INST]: Explain why free electrons in an insulator cannot jump over the energy gap to the conduction band [/INST]: Free electrons in an insulator cannot jump over the energy gap to the conduction band because of the energy barrier that exists between the valence and conduction bands. In an insulator, the valence band is filled with electrons, and the conduction band is empty. The energy difference between the valence and conduction bands is known as the bandgap. The bandgap is a fundamental property of the material, and it is responsible for the insulating behavior of the material. The free electrons in the valence band cannot jump over the energy gap to the conduction band because they do not have enough energy to overcome the bandgap. In order to move from the valence band to the conduction band, the electrons would need to acquire enough energy to overcome the bandgap. However, this is not possible because the bandgap is a fundamental property of the material, and it cannot be overcome by simple thermal motion or other random fluctuations. Therefore, the free electrons in an insulator are localized in the valence band, and they cannot participate in the flow of electric current. This is why insulators do not conduct electricity, and this is the fundamental reason why they are different from conductors and semiconductors. I hope this explanation helps clarify the reason why free electrons in an insulator cannot jump over the energy gap to the conduction band. If you have any further questions or need additional clarification, please don't hesitate to ask. [INST]: /stats prefill: 2.4 tok/s, decode: 1.2 tok/s |
ซึ่งในขณะที่ระบบทำการประมวลผลโมเดล Llama-2-13b-chat-hf-q4f16_1 จะใช้ RAM อยู่ที่ 10.6G
ผลการทดลอง
จากผลการทดลองด้วยการใช้ prompt เดียวกันสอบถามไปยังโมเดลแต่ละตัว โดย prompt คือ “Explain why free electrons in an insulator cannot jump over the energy gap to the conduction band”
ทุกโมเดลสามารถให้คำตอบจากคำถามนี้ได้หมด แต่ว่าแต่ละตัวใช้เวลาในการมวลผลที่ต่างกันโดยเมื่อจบคำตอบเราจะดูสถิติว่า decode ได้เร็วช้าแค่ไหนจากค่า tok/s ซึ่งเป็นอัตราการประมวลผลโทเค็น (คำหรือหน่วยย่อยของคำ) ของโมเดล ต่อวินาที ซึ่งได้ผลตามตารางดังนี้
Model | Prefill (tok/s) | Decode (tok/s) |
---|---|---|
RedPajama-INCITE-Chat-3B-v1-q4f16_1 | 4.6 | 5.1 |
Llama-2-7b-chat-hf-q4f16_1 | 4.8 | 2.8 |
Llama-2-13b-chat-hf-q4f16_1 | 2.4 | 1.2 |
หลังจากนั้นเราจะทำการตรวจสอบความถูกต้องของคำตอบ เพื่อให้ได้ความเห็นที่เป็นกลางเราเลยทำการโยนคำตอบทั้งหมดไปยัง Google Gemini ให้ช่วยออกความเห็นว่าคำตอบไหนดีที่สุด
ความเห็นจาก Google Gemini
1 2 3 4 5 6 7 8 9 10 11 12 13 14 15 16 17 18 19 20 21 22 23 24 25 26 27 28 29 30 31 32 33 34 35 36 37 |
Here's my evaluation of the three answers you provided: Answer 1: Strengths: Accurately describes the concept of the energy gap and its role in conductivity. Mentions the difference between valence and conduction bands. Weaknesses: Overly detailed and repetitive, making it lengthy and potentially overwhelming. Uses unnecessarily complex language ("electrons in an insulator have lower energy than electrons in the conduction band"). Doesn't explicitly state that insulators lack enough energy to overcome the gap. Answer 2: Strengths: Polite and friendly introduction. Clearly points out the misconception in the question. Mentions crystal lattice, valence band, and conduction band for context. Weaknesses: Slightly indirect approach to answering the question. Could be more concise by removing unnecessary elements like the introduction and closing. Answer 3: Strengths: Well-structured and concise explanation. Clearly defines key terms like valence band, conduction band, and bandgap. Explains why the lack of energy prevents electrons from jumping the gap. Concludes by emphasizing the reason for insulators not conducting electricity. Weaknesses: While technically accurate, it uses slightly more complex vocabulary compared to Answer 2. Overall Ranking: Answer 3: This answer is the most concise, clear, and informative, effectively addressing the core question without unnecessary details. Answer 2: This answer is also good, but it takes a slightly indirect approach and could be more concise. Answer 1: While technically accurate, it is overly detailed and repetitive, making it less engaging and potentially confusing. |
ซึ่ง Google Gemini ให้คะแนนคำตอบจากโมเดล Llama-2-13b-chat-hf-q4f16_1 สูงที่สุด ตามมาด้วย Llama-2-7b-chat-hf-q4f16_1 และ RedPajama-INCITE-Chat-3B-v1-q4f16_1 เป็นลำดับสุดท้าย
สรุปผล
จากการทดลองใช้งานในด้านที่เกี่ยวข้องกับ AI พบว่าบอร์ด Mixtile Blade 3 ทำได้ดี ให้ประสิทธิภาพการทำงานที่ดี ด้วย RAM ขนาดความจุสูงสุดที่ 32 GB รองรับการใช้งาน AI โมเดลขนาดใหญ่ได้เป็นอย่างดี และด้วย eMMC ขนาดความจุสูงสุดที่ 256GB และยังรอบรับ NVMe ทำให้สามารถต่อ SSD ได้รองรับการเก็บข้อมูลได้อย่างไม่ต้องกังวล
เคส Mixtile Blade 3 งานผลิตถือว่าคุณภาพดีมาก มีการออกแบบให้รองรับการใช้งานไร้สายโดยไม่มีการลดทอนสัญญาณ เพราะมีการออกแบบส่วนประกอบพลาสติกร่วมกับอลูมิเนียมได้อย่างกลมกลืน แต่การทดสอบใช้งานพบว่าเสียงพัดลมดังมากและเคสค่อนข้างร้อนแม้จะใช้งานในห้องทีมีเครื่องปรับอากาศ
ในส่วนของเอกสารประกอบการใช้งานทำได้ดีครบถ้วน จัดวางเนื้อหาเป็นสัดส่วนที่ดี ทำให้ผู้ใช้สามารถใช้งานได้โดยไม่ยุ่งมากมีวิดีโอประกอบให้ทำตามได้อย่างง่ายดาย
แต่การใช้งานจะพบว่าการเชื่อมต่อทำได้ค่อนข้างลำบาก หากต้องการใช้งาน Wi-Fi ต้องต่อผ่านคอนเน็คเตอร์ mPCIe หรือต่อจอภาพต้องใช้อุปกรณ์ต่อพ่วงเพื่อให้ใช้งานได้ เนื่องจากการที่มีพอร์ต USB C เพียงสองช่อง
แต่หากมองภาพการนำไปใช้งานเฉพาะด้าน เช่น cluster computer ถือว่ามีความคุ้มค่ามาก เพราะบอร์ดออกแบบมารองรับการใช้งานด้านนี้ไว้แล้วสามารถต่อเชื่อมเป็นคลัสเตอร์ได้อย่างง่ายดายพร้อมซอฟต์แวร์ไดรเวอร์
ต้องขอขอบคุณ Mixtile ที่ได้ส่ง Mixtile Blade 3 มาให้เราได้รีวิว และหากสนใจที่จะซื้อบอร์ด Mixtile Blade 3 มาเพื่อใช้งานสามารถสั่งซื้อได้ที่ช่องทางต่าง ๆ ดังนี้ บนร้านออนไลน์ Mixtile shop ราคาเริ่มต้นที่ $229.00(~8,200฿) สำหรับ RAM 4GB และ Storage 32GB, สูงสุด RAM 32GB และ Storage 256GB ราคา $439(~15,700฿) และบน Aliexpress ราคาเริ่มต้นที่ $352(~12,700฿) ขึ้นอยู่กับ RAM และ Storage ที่คุณเลือก
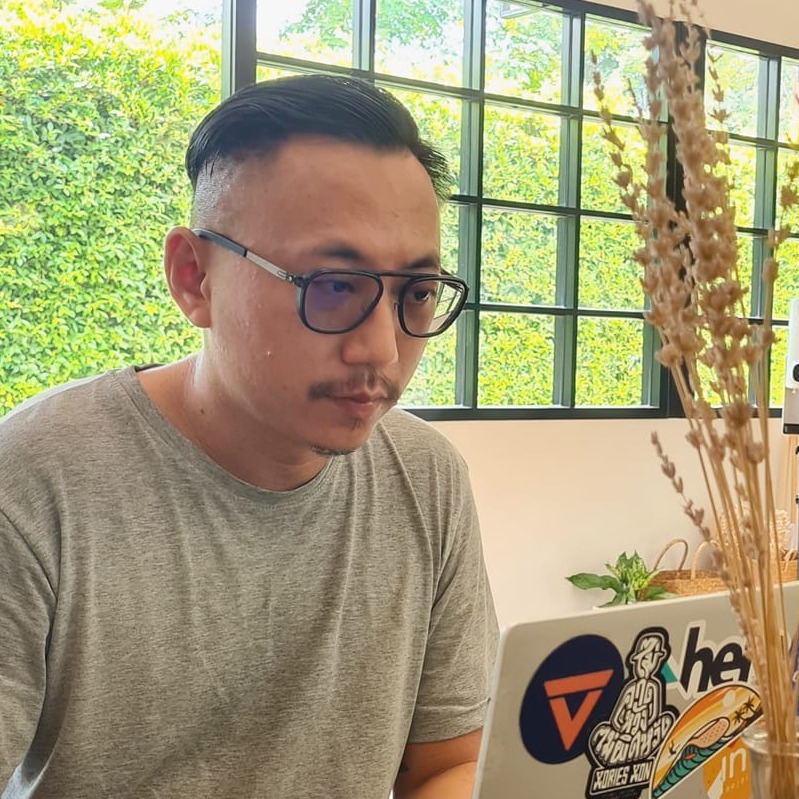
Fulltime Maker
ปัจจุบันดูแลส่วนงาน R&D และ Innovation
ความสนใจคือ Single Board Computer และงานด้าน IoT